Data in Motion - The evolution & future of data engineering
Forget static dashboards and stale reports—data doesn’t sit still anymore. In this high-velocity premiere of Beyond Next, Arun Kumar and Vipul Valamjee unravel what it really means to engineer data in motion. From self-healing pipelines and real-time analytics to AI-powered architectures and the rise of semantic layers—this episode is a front-row seat to the future of data.
If you think data engineering is just about rows and columns, think again. This is where business, AI, and innovation collide—and nothing stays still for long.
Podcast transcript
🎙️ Podcast Title: Beyond Next
📌 Episode Title: Data in Motion: AI, Real-Time Pipelines, and the Future of Data Engineering
🎧 Hosted by: Arun Kumar
🎤 Guest: Vipul, Expert in Data Engineering and Management
[Intro Music]
Arun Kumar:
Welcome to Beyond Next, Ultimatric's own podcast on all things digital. I’m your host, Arun Kumar, and today we have an exciting discussion with Vipul, a leading expert in data engineering and management.
As we all know, AI is the buzzword today. Every enterprise is trying something new with AI, and conversations around artificial intelligence and machine learning are increasingly focused on the value they can create for businesses. But at the foundation of all these innovations lies data—it's truly the cornerstone.
In this episode titled "Data in Motion", we’re going to explore the latest trends, innovations, and challenges in the world of data.
With that, Vipul, welcome to the show.
Vipul:
Thank you, Arun.
The Evolution of Data Engineering
Arun:
Vipul, you and I have been involved in many engagements where the very construct of data engineering has changed significantly over the last 4 to 5 years. Can you walk us through some of the biggest transformations?
Vipul:
Sure, Arun. Data engineering has evolved dramatically over the years—from simple flat files and RDBMS systems to data warehouses, and now to modern data lakehouses, which have become the norm.
For AI to work efficiently, it needs data in all forms—structured, semi-structured, and unstructured. This is where lakehouses come into play. They allow AI models to directly access unstructured data, cutting down on data conversion time.
Also, the pipelines themselves have become intelligent. Today’s pipelines can auto-detect changes and adjust without manual intervention. This fundamentally accelerates how AI can derive value from data and makes the entire ecosystem more scalable and responsive to business needs.
Real-Time Data Processing
Arun:
That’s a good segue into real-time data processing—especially with unstructured data. What does real-time processing look like today?
Vipul:
Historically, real-time meant ingesting data from IoT or transactional systems, transforming it into structured formats, and then analyzing it.
Now, real-time is truly real-time. Instead of converting unstructured data into structured formats first, AI models can directly tap into unstructured data. This drastically reduces latency.
With AI-driven ingestion, data can be consumed and acted upon instantly, whether it's in JSON, XML, video, or even audio formats. This capability has significantly changed how we think about real-time analytics.
AI’s Role in Data Management
Arun:
Let's now shift to how AI is changing the way we handle data itself.
Vipul:
Absolutely. Traditionally, we had three major components:
- Data engineering – for pipeline development
- Data management – for MDM, reference data, quality
- BI or analytics – to consume and visualize data
With AI, especially conversational AI, we’re seeing a transformation. Enter the semantic layer—this acts as a bridge between business terminology and technical data fields.
Imagine a CXO asking, “What are my top 10 products by revenue this month?” The semantic layer converts this into a SQL query, executes it, and returns the result—all in natural language. It reduces the need for heavy engineering intervention and enables business users to directly interact with data.
AI-Driven Security and Compliance
Arun:
Now let’s touch on security. With increasing data flows, how is AI helping with data protection and compliance?
Vipul:
Great question. AI in security isn’t new—fraud analytics has been around for over a decade. We’ve moved from simple rule-based systems to AI-powered anomaly detection and threat identification.
Now, systems use historical patterns to not just flag threats but also take automated action in some cases.
Of course, regulations like GDPR, DPDP 2024, and CCPA require human oversight to define the compliance rules, but once those rules are in place, AI can monitor and enforce them effectively in real-time.
The Future of Data Engineering
Arun:
Where do you see data engineering headed in the next 5 to 10 years?
Vipul:
The future is autonomous, AI-optimized, and performance-tuned.
We're already seeing self-healing databases and AI-first architectures like Oracle Autonomous Database or Snowflake AI. Data engineers will now focus more on:
- Performance tuning using metadata
- Optimizing data models dynamically
- Building data pipelines that can fix themselves
And most importantly, AI-driven metadata generation will remove a lot of manual effort in understanding and mapping data.
The Rise of DataOps and MLOps
Arun:
Let’s go deeper into DataOps and MLOps. What are you seeing there?
Vipul:
DataOps is about automating data workflows. Tools like Monte Carlo and Datafold offer real-time observability, minimizing business disruptions.
MLOps, on the other hand, focuses on getting AI models into production efficiently. We're moving from 90% of ML models never reaching production to almost 40% being production-ready today.
MLOps ensures:
- Model monitoring
- Data drift detection
- Automated re-training
- Continuous accuracy tracking
This is essential, especially as more businesses become dependent on AI models for daily operations.
Implementing an AI-Driven Data Strategy
Arun:
So, if a company wants to adopt an AI-driven data management strategy—where should they start?
Vipul:
Great question. Here’s a five-step roadmap we usually recommend:
- Assess the architecture – Identify bottlenecks in infrastructure and pipelines.
- Intelligent ingestion – Build or upgrade pipelines that can auto-detect and fix data issues.
- Semantic layer integration – Start small using BI tools like Power BI or Tableau, which already offer semantic capabilities.
- Secure and govern – Use AI-driven security tools to manage compliance and detect anomalies.
- Adopt DataOps and MLOps – Automate and monitor the full data-to-AI lifecycle.
Start small, with 2-3 high-impact use cases, then scale from there.
Final Thoughts
Arun:
Vipul, any final thoughts?
Vipul:
Yes—AI and GenAI are not just buzzwords anymore. They’re fundamentally changing how data is managed, stored, and used.
Start small, think big. Focus on specific business use cases, build the pipeline from ingestion to insights, and scale from there. The future of data is autonomous, AI-driven, and business-aligned.
Arun:
That’s a wrap! Vipul, thank you for the incredible insights—it’s been a fantastic discussion.
And to our listeners, if you enjoyed this episode, don’t forget to subscribe and stay tuned for more thought leadership from Beyond Next by Ultimatric.
Until next time—goodbye!
Vipul:
Goodbye, everyone!
[Outro Music]
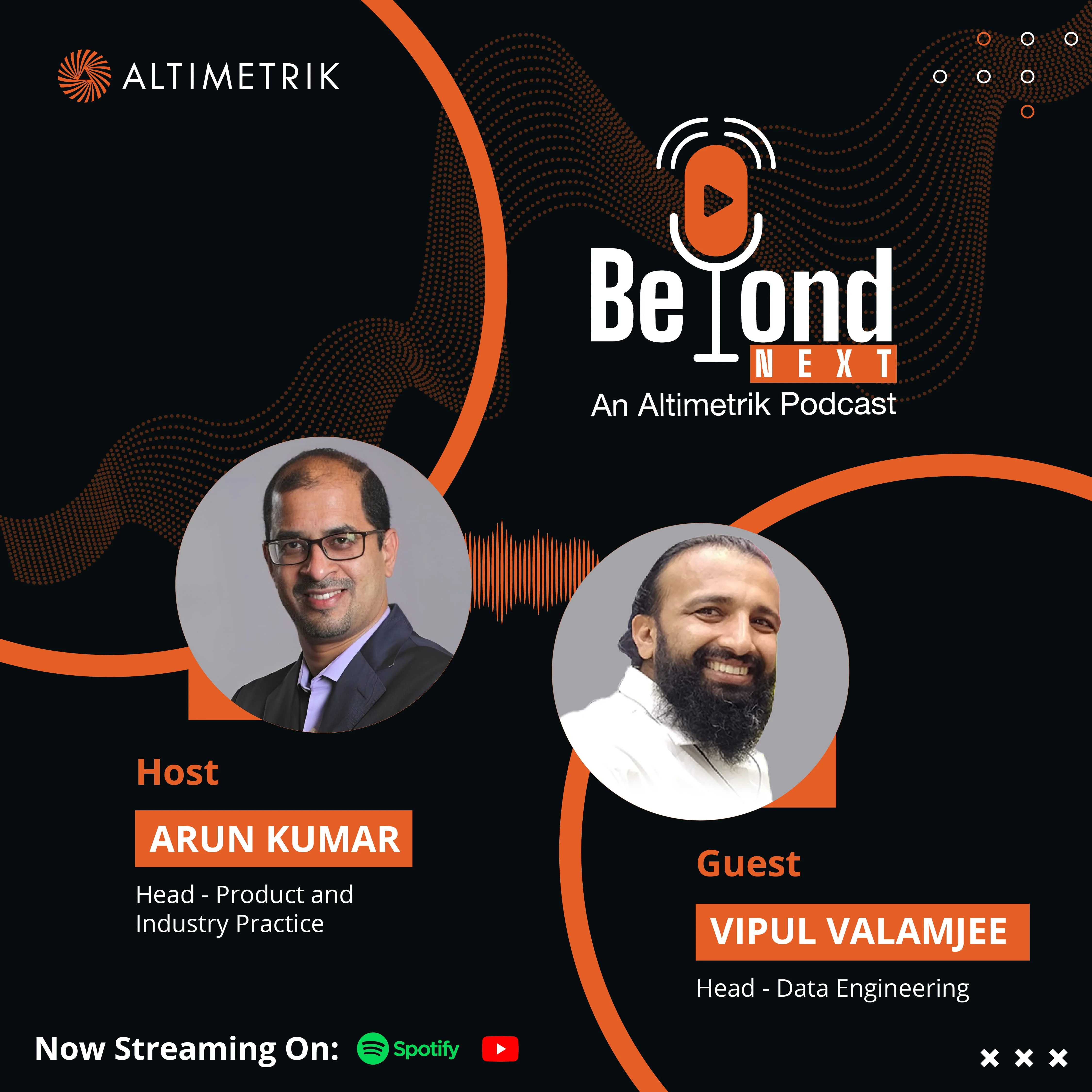