What is Big Data? Untangling Data Terminology for Digital Success
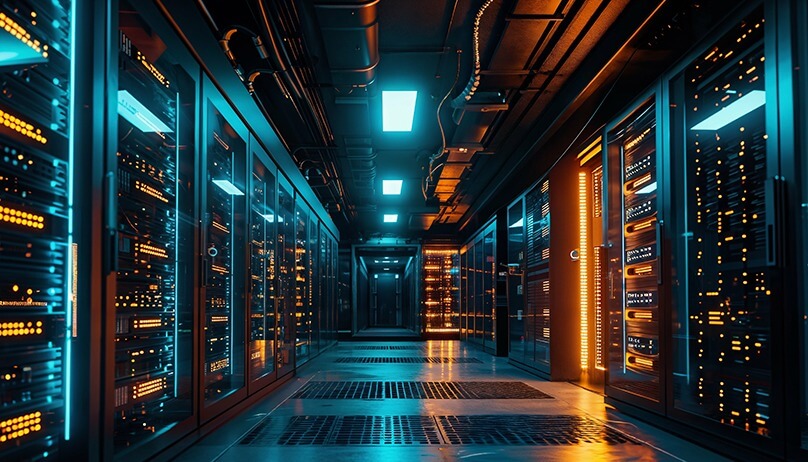
Demystifying Data Terminology
With global spending on big data and business analytics forecasted to increase 10.1% this year, most every enterprise across every industry is gearing up for its own data initiatives
This trend will only continue over the next five years, according to IDC, as the global economy recovers from the COVID-19 pandemic. Data is on everyone’s mind — how to find it, connect it, interpret it, use it, and protect it.
Still, we’ve repeatedly seen customers express confusion over basic terms in the data/analytics domain. Many terms are wrongly used interchangeably, and when the terminology is misused or misunderstood in meetings where big decisions are made, these casual slips can cost millions.
Take the phrases big data, data analytics, and data science, for example. A Google search reveals 160 million results for big data, nearly three times more than data analytics or data science. Why such a discrepancy? Big data makes for catchier headlines and has surely generated more hype because of its potential, but too often we see it misused or misapplied to data initiatives large and small. That’s just one reason why most big data science/analytics projects fail to produce business outcomes.
The pace of data is accelerating all around us, so there’s no better time to review basic data terminology and how it enables your digital initiatives.
What Exactly Is Big Data?
what is big data? Big data is a bit of a misnomer. It’s not just any data that is too big (a volume problem), it’s also data that’s too fast (a velocity problem), or has too many varieties of structured, unstructured, or semi-structured data (a variety problem) for traditional databases to handle. Volume, velocity, and variety represent the original “Vs” of big data. Veracity (quality) and value (ability to transform data into business) have since followed as our ability to harness more data faster became more powerful.

So it’s not just about volume. If you have a small amount of data flowing in at a fast pace and you need an automated system to analyze and get insights from it in real time, you’ve still got a big data problem despite the data itself being small.
Now, if you’re talking about big data analytics, it would be incorrect to say it’s complex and small data analytics is not. Consider a scenario in which you’re given sales data for the last three years and asked to forecast sales for the next five weeks. With only 156 rows of data (52 weeks x 3 years), you wouldn’t have enough to confidently draw a reliable conclusion. So here the data is not big, but the analytics are still challenging.
So then why all the hype around big data analytics? Part of it is just that — hype — since you can indeed perform advanced analytics even using data small enough to be contained in a common XLS file.
But part of it is not hype. Big data ecosystems — both the traditional Hadoop-based architectures, as well as modern cloud-based data systems, enable more data to be assembled at a much lower cost than before. This creates more opportunities for identifying valuable use cases for insights — think of it as having a much larger playground in which to create new revenue opportunities.
How Data, Information, Knowledge, and Insights Create Business Value

Data means different things to different people. But in the realm of business analytics, it means raw data, like a customer’s zip code, say 90210. The number by itself doesn’t mean much, and the presentation of the raw number or statement — “the customer lives in 90210” — begs the question, “So what?”
Information is an inference we make from the raw data. “The customer lives in Beverly Hills, California.” While this adds some color and context to the conversation, there is still an opportunity to ask, “OK, so what?”
Knowledge is what helps create a decision based on past experience, heuristics, rules, and the information at hand. “We will send this customer offers for luxury items” because we know Beverly Hills is one of the most affluent cities in the country.
The business value typically increases as you climb the ladder from data to information to knowledge. It’s the job of a data analyst or data scientist (they are different, too) to take the data and extract information and knowledge. Information and knowledge are the insights used to make business decisions, shaping marketing tactics or communications preferences.
Do You Need a Data Engineer, Data Analyst, Or Data Scientist (Or All Three)?
A data engineer ingests data from different data sources, and transforms, cleans and prepares the data for analysis. It’s also the data engineer’s job to prepare and maintain a Single Source of Truth (SSOT) to ensure harmonious work across business functions. So when you hear SQL Server, Oracle, Hadoop, Data Lake, Data Warehouse, SQL, Spark, PySpark, Cloud Data Services (like AWS Glue, AWS Lambda, Azure Data Lake), think ‘data engineer’.

A data analyst primarily uses SQL and BI/visualization tools for getting insights from the data. So when you hear Tableau, Qlik, PowerBI, Spotfire, Metabase, Superset, or SQL, think ‘data analyst’.
A data scientist also extracts insights from the data, but this person needs to use a broader/deeper repertoire (aka “advanced analytics”) than a data analyst. So when you hear Python, R, SAS, SPSS, Machine Learning, AI, or Neural Networks, think ‘data scientist’.
One could say that a data engineer writes data into the SSOT, and then a data analyst or data scientist reads data from the SSOT. Again, an oversimplification, but you get the larger picture.
In terms of responsibilities, the SSOT typically acts as the hand-off point between the data engineering team and the data analytics/science team.
In terms of architecture, the SSOT typically acts as the abstraction layer between the data architecture and the analytics architecture, providing a separation of concerns.
In terms of organization, data engineering is typically a part of the IT/engineering organization. Traditionally, the data science teams were sponsored more by the business function, but nowadays you see them in both IT/engineering as well as the business side.
How Does Data Governance Fit In?
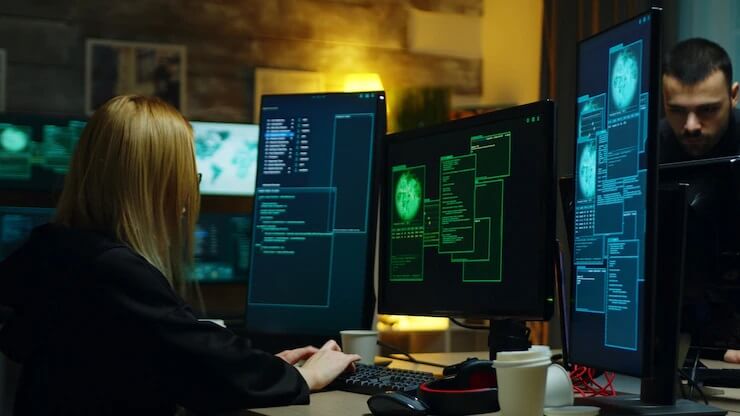
Data governance is a broad area consisting of data quality, master data management, lineage/provenance, and security. This is the discipline that ensures that the data ingested using data engineering pipelines is converted to, and maintained in, a state that is useful for extracting reliable insights. Otherwise the risk is “garbage in, garbage out.” Data governance falls under the ambit of the data engineering team as it prepares the SSOT.
Occasionally, you may hear the term data management. That’s basically a combination of data engineering pipelines plus data governance – the “write to SSOT” side. And when you hear the phrase “data & analytics,” data implies management, while analytics implies data analysis/science.
The Right Terms Yield Results
Understanding data terminology will only grow in importance as data’s impact expands. Building the right vocabulary for internal and customer discussions will enable greater collaboration to gain real-time insights that enable better decision-making. It will also position enterprises to connect customer identities, marketing funnels, supply chains, and data privacy and manage all the moving parts more efficiently.