Digital Twin – A Potential Game Changer for Drug Development

The concept of Digital Twins is being actively investigated by Pharma companies. This technology has great promise as one of the levers to modernize the pharmaceutical industry. A recent digital health technology report states that 66% of pharma organizations are planning to invest in digital twins in the upcoming years. According to Markets and Markets research, the digital twin market is expected to reach 73.5 billion by 2027 growing at a CAGR of 60.6% (2022-2027).
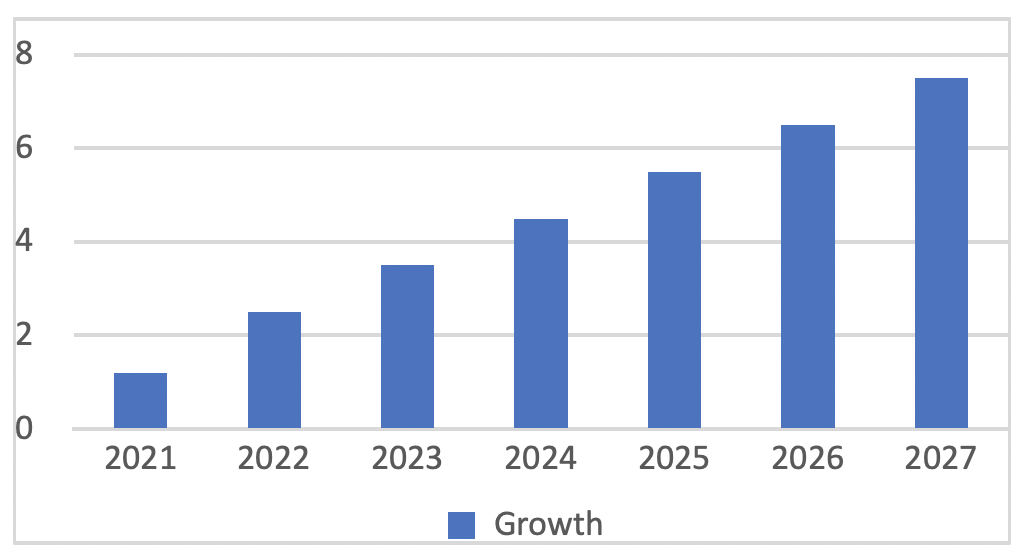
What is a Digital Twin?
A digital twin is a virtual representation of a physical asset, process, or system that allows for real-time monitoring, analysis, and optimization. It is created using data from various sources, such as sensors, electronic health records, and imaging technologies, and can be used to simulate the behaviour of the physical counterpart.
How does it apply to the pharmaceutical industry?
In the pharmaceutical industry, a digital twin is a virtual representation of a drug, process, or system that is used to optimize the drug development process. As mentioned in the above statement digital twins can be created using data from various sources, including electronic health records, wearable devices, and imaging technologies. In the pharma industry, digital twins can be used to simulate the behaviour of a drug in the body, allowing researchers to better understand its potential side effects and tailor the dosage and administration accordingly. This can lead to more personalized and effective treatment for patients. This can help identify bottlenecks or potential issues before they occur in the physical process, saving time and resources in the life sciences industry.
Some of the Key Challenges in the Clinical Trial Process
Recruitment and retention of study participants: It can be challenging to find and enroll enough qualified study participants, and to keep them engaged and compliant with the study protocol, especially for rare diseases or conditions. Recruiting and retaining patients for clinical trials has always been a barrier. Currently, 85% of all clinical trials fail to recruit enough patients, and 80% are delayed due to recruitment problems.
Data collection and management: Compared to traditional trials, decentralised clinical trials generate large amounts of data that must be collected, managed, and analyzed accurately and efficiently. This can be a complex and time-consuming task, particularly when dealing with large amounts of data from multiple sources.
Multiple site management: It can be challenging to design a clinical trial that is scientifically rigorous, ethically sound, and practical to implement. Clinical trials occur in multiple sites thus increasing the number of sites, participants, and vendors leading to complex situations or circumstances.
Regulatory approval: Clinical trials must adhere to strict regulatory guidelines to ensure the safety and effectiveness of new drugs and treatments. This can involve navigating complex regulatory processes and complying with various requirements for the conduct of clinical trials.
Ensuring the safety of participants: Clinical trials must follow strict ethical guidelines to ensure the safety and well-being of participants. This can involve obtaining informed consent from participants, protecting their privacy, and minimizing any risks to their health.
Quality control: Ensuring the quality and reliability of data collected during clinical trials is critical to the success of these studies. This can involve implementing strict quality control measures to ensure that data is collected and analyzed accurately and consistently.
Some medtech companies are working with digital twins, but they face several challenges in accessing patient data due to privacy and security concerns, issues related to data ownership, challenges in ensuring data quality and completeness, and the presence of data silos.
What the future looks like
Traditional clinical trials are now moving towards decentralised clinical trials that are conducted remotely or outside of traditional research settings. Digital twins in decentralized clinical trials can simulate the trial environment and assess feasibility. They can simulate drug distribution, data collection, and communication between participants and staff. This helps identify challenges and opportunities related to decentralization and develop strategies for addressing them. Digital twins may provide a useful tool for optimizing trial design and improving efficiency and effectiveness.
Pharma companies can adopt data technologies such as machine learning, analytics, and cloud computing to leverage digital twins more effectively. These technologies can be used to improve the accuracy and reliability of digital twin simulations, and to process and analyze large amounts of data generated by digital twins.
Additionally, pharma companies can use data technologies to integrate digital twins with other systems and processes, such as supply chain management, manufacturing, and regulatory compliance. By adopting these technologies, pharma companies can gain a deeper understanding of their products and processes and use digital twins to optimize their operations and improve their decision-making.
Stay tuned for more on the ways advanced technology and analytics impact clinical trials and their usage which is showing great promise to the pharma industry.